Evróputilskipun um gagnsæi í launum | Sækja rafbók hér.
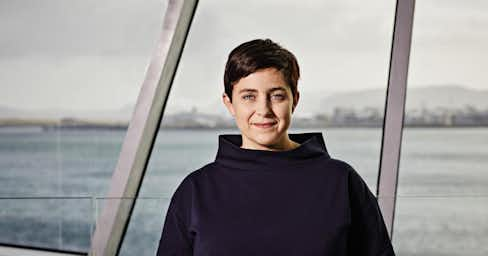
People analytics as a tool to improve pay equity — a blog post for Trend Report
In a recent blog post for the German HR magazine Trend Report, PayAnalytics Co-founder Margrét Bjarnadóttir explained how important it is to recognize the limitation of AI-based HR tools when making important decisions about hiring, compensation, promotions, and training opportunities. Even when workplaces do strive for equity, their data can still reflect historical biases and discriminate on the basis of ethnicity, gender, and class. Hence, to improve pay equity, companies need to find the right tools, identify where there are potential biases, and take the necessary steps to eliminate those biases.
People analytics as a tool to improve pay equity
Wage inequality is a pressing issue that has been exacerbated by the pandemic. Millions of women have dropped out of the workforce and many people have changed jobs, either through internal moves or by switching employers. People analytics, the field that applies quantitative tools to the employee value chain, can support organizations in realizing pay equity and building more equitable workplaces. However, these tools require a thoughtful application, as even when workplaces do strive for equity, their data can still reflect historical biases and discriminate on the basis of ethnicity, gender, and class. Hence, to improve pay equity, companies need to find the right tools, identify where there are potential biases, and take the necessary steps to eliminate those biases.
Recognizing the limitations of AI-based tools
Today, AI-based people analytics tools are used to help managers measure productivity and make important decisions about hiring, compensation, promotions, and training opportunities. Many companies also use this technology to identify and close pay gaps related to gender, ethnicity, or other key demographic categories. As this use of AI in HR increases, however, it is important that its deployment and use are monitored to ensure that systems are robust, fair, and secure. These systems are only as good as the data and assumptions underlying them, so it is critical to ensure that the data is high-quality and reliable. Further, it is vital to understand the consequences and implications of the technology. Given the complexity and scale of the tasks usually delegated to AI, there is a real risk that models will go wrong. Without the necessary guidance and proper training, AI can churn out answers that lead to unknowingly biased decisions with potentially damaging consequences.
This means that first, employers need to understand that data is not neutral and can contain bias. People analytics tools are generally built off an employer’s historical data on the recruiting, retention, promotion, and compensation of its employees. Such data captures the decisions and attitudes of the past. Managers must therefore remain aware that their retrospective data may reflect both old and current biases and may not fully help them address the complexities of people management in an increasingly diverse workforce. For instance, grades might be used as a proxy for intelligence, or occupational licenses or certificates may be taken as a measure of skills. However, these measures are incomplete and often contain biases and distortions. Thus, when applying any people analytics tool, it is important to consider the potential mismatches between what companies want to measure (e.g., intelligence or ability to learn) and what they actually measure (e.g., performance on scholastic tests).
Additionally, companies need to understand that a people analytics tool’s performance is a product of both the data it is fed and the algorithm it uses. Hence, managers should consider that models are likely to perform best with individuals in majority demographic groups but worse with less well represented groups. This is because algorithms typically maximize overall accuracy, so performance for the majority population carries more weight than performance for the minority population in determining algorithm parameters. Also, there is no such thing as a truly “ethnicity-blind” or “gender-blind” model, and explicitly omitting ethnicity or gender from a model will not make the models “demographic neutral” – in fact, it can even make things worse. Moreover, if demographic categories are not evenly distributed throughout the organization, which is almost always the case, even carefully built models will not lead to equal outcomes across groups. Hence, to avoid making biased decisions, people in charge need to pay close attention to who is represented in the data, remain especially aware of the limitations of AI-based models, and monitor the models’ application across demographic groups.
Detecting bias reliably with various tools
To ensure that they people analytics tools deliver useful beneficial decision support , organizations can use a variety of tools. One particularly helpful tool is a bias dashboard. A dashboard summarizes how the people analytics tool performs across different groups, allowing for early detection of potential bias. It highlights both statistical performance and the tool’s application impact across groups. For example, in a hiring support application, the dashboard can summarize the potential applicants “poked” on different sites (e.g., LinkedIn) as well as the proportion of each group that applied, received an interview and was ultimately hired.
In addition to monitoring statistical performance metrics, managers can also explicitly test for bias. For example, they can study how individual features, such as experience or training, contribute towards pay increases for different groups. They can also study the outcomes of the annual review process. For instance, if employees of one ethnicity are disproportionately recommended for a salary increase, this is a sign that the AI tool or the decision process may be biased. In this case, following the tool’s recommendations would reinforce the bias. It could also be that the tool is disproportionately identifying minorities as candidates for raises because they tend to be underpaid in the company, in which case the AI tool is helping the company solve the problem.
It is important to remember that no model is complete. For example, an employee's interpersonal skills may influence their success within the company without necessarily showing up in the employee's HR data. The users of people analytics tools need to be aware of these additional factors and learn how to systematically include them in the decision making process. If there is quantifiable data related to these factors, they should consider collecting it. Also, any time an AI algorithm is used for decision making, the company needs to be able to explain to all stakeholders what that result means and how it was arrived at. Algorithms can help interpret past data and identify patterns, but people analytics is still an area where humans are central to decision making – so the final decisions, and the responsibility for these decisions, rest with the humans
Outlook
People analytics tools, especially those based on AI, have become indispensable in modern HR; however, quantitative models are intended to assist, not replace, human judgment, companies must remain aware of machine learning biases and model limitations. To get the most out of AI and other people analytics tools, companies will need to consistently monitor how the application is working in real time, what explicit and implicit criteria are being used to train the tool, and whether the outcomes of the tool’s recommended decisions affect different groups differently in unintended ways. In order to harness people analytics appropriately and create truly equitable workplaces, managers must learn to ask the right questions about their data, their decisions, their quantitative models, and their software.